Are You Ready For AI Agents? A CTO’s Perspective
What’s the next big thing for AI? In my previous blog, I looked back over the last year or so to uncover key learnings on successful AI implementation. This time around, I’m looking ahead at AI agents – software that’s able to perform tasks autonomously.
If you’ve ever used an online customer services chatbot, driven a self-parking car, played a video game with NPCs (non-playable characters), or used an AI assistant, then you’ve interacted with an AI agent.
But is your organization ready for an AI agent of its own? Let’s find out.
By Martin Nürnberg Gundertofte, CTO, WorkPoint
AI agents can do more for your organization
At the moment, AI can reliably handle straightforward tasks – like requests for information or answering simple queries within a customer services workflow – before handing users over to a human agent. On a basic level, the AI dives into a system, extracts relevant information, and presents it to the user.
But it’s interesting how AI agents have evolved in the last year or so. Prompts that were once met with ‘I’m unable to do that’, are increasingly possible. And no wonder, the big tech companies have invested heavily in AI tools. They’re pushing an obvious agenda here; they want you to use their AI in your everyday activities. We’ve started to see the beginning of this with the roll-out of Copilot. For that reason, in the coming years, we can expect AI agents to have deeper and deeper integration inside ecosystems like Microsoft 365.
Not only does this represent a shift in how AI technology is deployed, it also asks people to make a leap of faith, and put greater trust in AI. As I previously discussed, we’re at the stage where, with appropriate verification and validation checks in place, we increasingly trust the information delivered by AI. Now we’re being asked to trust that an AI can perform autonomous actions on our behalf.
The next step involves entrusting AI to perform more complex tasks. Right now, no AI model is 100% accurate. If you prompt an AI model with a question, there is a certain level of uncertainty, and therefore risk. It’s up to individual organizations to decide what constitutes an acceptable level of risk and margin of error. In many cases, although the uncertainty is very low, the impact of an error is considered an unacceptable risk to the business.
For example, if a response from an AI customer service chatbot is wrong, the relevance and quality of the next response will decline, and snowball. From a financial perspective, if the entire process could be smoothly handled by a human agent, investment in an AI agent may not result in any meaningful ROI: yet.
Having an AI agent isn’t enough; does it add value?
Many organizations jumped on the AI bandwagon to boldly proclaim their products or services are enhanced with AI. Ultimately, like any software product, if your AI tool isn’t delivering value to your company or customers, it isn’t bringing anything to the table.
The same applies to AI agents. It’s important to remember that AI agents are designed to provide answers. As we’ve already discovered with publicly available chatbots, that sometimes prioritizes giving an answer, regardless of whether it’s factually correct. This means that ‘probably right’ answers are given over no answer at all.
Here’s a great example. You need the phone number for someone in a different company. You know their name, their role, and the company name. But the AI chatbot doesn’t have the correct number, so it keeps suggesting phone numbers that are simply incorrect.
What this relatively low-impact error highlights is that one of the best ways to get started with an AI agent is to trial it on a non-critical aspect of your business. As with any new technology, whatever your reasons for spending time and resources on it, the key question is always: how does it add value?
One of the key learning points from the past year or so is that organizations need to know if they’re getting tangible value from AI tools, or whether they’re simply investigating the potential value. Until there’s a viable way to measure the value of AI, it’s difficult to have a conversation about ROI.
Ultimately, if you want to start depending on an AI agent for a semi-critical aspect of your business, ‘probably right’ probably isn’t good enough.
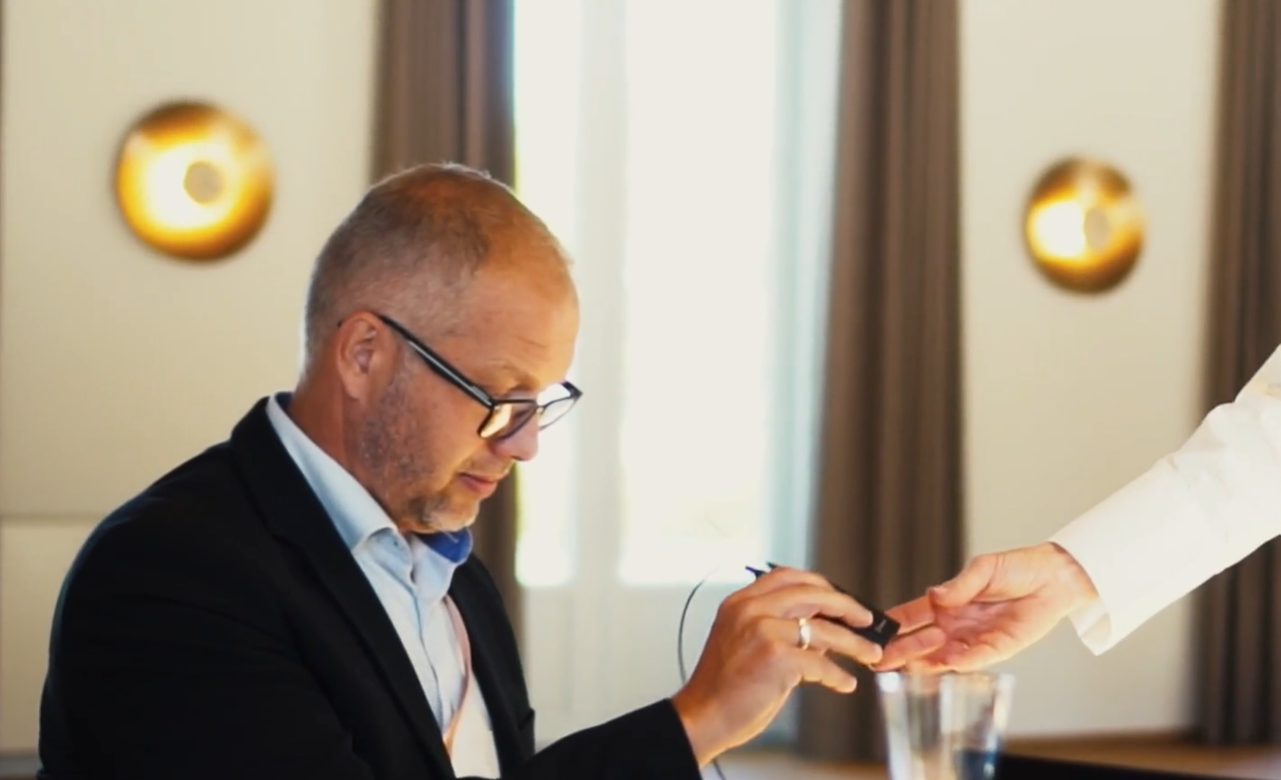
Coding AI agents takes time and resources
Right now, there are lots of people claiming to be an ‘AI specialist’. But what does that mean, exactly? AI implementation is still in its early stages, so finding a genuine expert isn’t easy. While there are toolkits available for building AI agents, the reality is that it takes deep technical expertise and resources to develop something that can truly add value to your business. For instance, developing an AI agent that incorporates decision trees is actually highly complicated to do effectively and reliably.
What we see from working closely with our WorkPoint Partners and customers is that many more businesses have now identified that AI can bring value to their operations, and they are currently in the process of defining concrete use cases. Many have experimented with AI to see how it can bring value, but the challenge is how to narrow it down to a specific use case.
For instance, let’s say a legal department working with contracts would like an AI tool to make contract creation much easier. They’d like to generate new contracts based on existing ones. Identifying this specific use case is only half the solution. The other half is finding the technical expertise to make this a reality, which in the emerging field of AI isn’t easy.
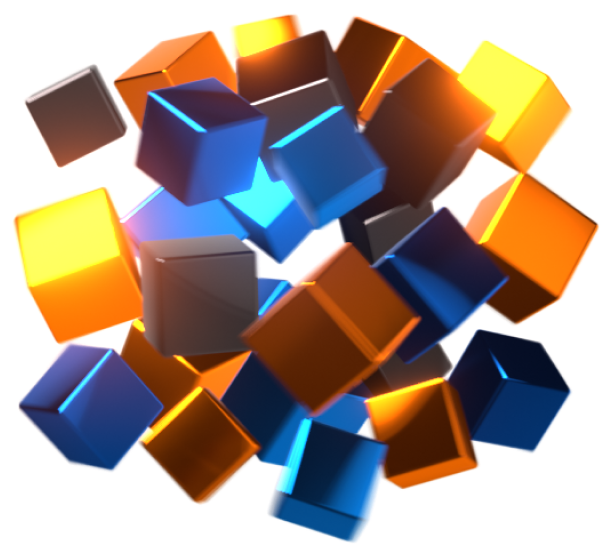
Data stewardship is vital for AI agents
A while ago, when I wrote about successful AI implementation, data stewardship was highlighted as a key aspect of good AI implementation. If the last 12 months or so have taught us anything, it’s that successful AI needs to be grounded in relevant data.
In many ways, feeding AI agents with outdated data is arguably even less effective than not having it at all. Let’s say 10% of your company data is from the last year, and the other 90% is older. If your AI model is grounded in outdated data – then, like an older GPT model – the responses you get from it will also be outdated.
Fine-tuning an AI model to optimise its output can be expensive and time-consuming to do, but you can keep the cost down by ensuring you train it on good data. For that reason, data stewardship and effective data governance are vital for getting high-quality outcomes from your AI agent.
Data security is important in every industry, but in areas like the public sector, where the information held belongs to citizens, it’s crucial that personal data is handled compliantly, in accordance with relevant regulations, such as the GDPR.
One potential use case is getting your AI agent to generate a project or case based on incoming information from an email. If there’s an error in the data, and it can easily be corrected, then there’s no problem. But if you had a critical case in the public sector, an error could lead to a data breach or adverse publicity, and the erosion of public trust.
Public authorities tend to be more cautious about implementing AI agents. For that reason, it’s highly likely that private sector organisations will be the trailblazers of AI agents, and the public sector will see a slower, more cautious, adoption rate.
AI is not the only tool in the tech toolbox
One of the pitfalls of AI implementation is when an organization tries to deploy it in scenarios where a simpler solution is still the most effective and viable. It’s easy to forget that AI is not a magic wand that will instantly take care of all your business inefficiencies. AI products and agents are expensive and time-consuming to build, and in some cases having a developer add a few lines of code or build in a small automation is all you need to drive efficiencies.
The message here is to use AI sparingly, in areas where you know it can have a valuable impact on your business. Especially, for CTOs, it’s important to remember that AI is just another tool in the box and that for some problems there may be simpler and more cost-effective solutions. When it comes to AI implementation, it’s important to be open-minded but critical – AI is not the answer to all your problems. At least, not yet.